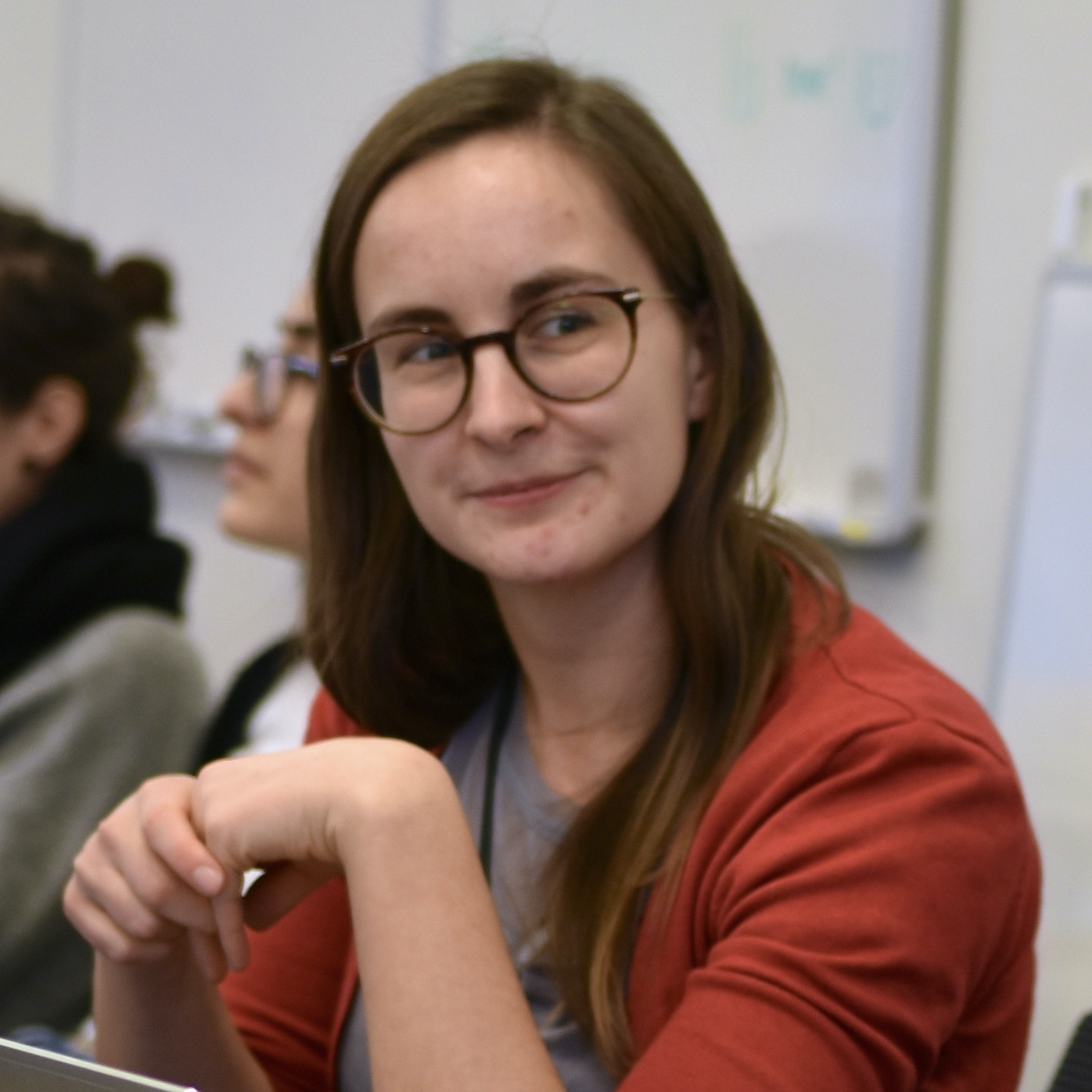
I’ve just finished reading a paper in a recent issue of Drug Safety called “Validating Intelligent Automation Systems in Pharmacovigilance: Insights from Good Manufacturing Practices”, which provides a lot of food for thought for everyone involved in medicines safety.
Pharmacovigilance relies on data, and as the field has expanded, not only has the science evolved rapidly, but the processes and practices of pharmacovigilance have led to an exponential growth in the number of case reports. The result of this growth is that the modern pharmacovigilance practitioner looks out upon mountains and mountains – and then even more mountains – of data. This is (a) great and (b) overwhelming.
So, over the years, the connections between pharmacovigilance and data science have become deeper and tighter. Some of the most exciting developments in pharmacovigilance now come from artificial intelligence (AI) and machine learning (ML), with new automated tools making it possible to mine those mountains, augment human intelligence, and enable ever more powerful and sophisticated analyses.
But there’s always a catch. In any field, increasing reliance on automation can come at the cost of transparency. With patient safety at stake, pharmacovigilance professionals cannot afford for their tools to be black boxes of unvalidated performance, where case reports go in one end and results mysteriously come out the other. They may be correct – or they may not. This paper addresses that problem by reviewing current validation methods and proposing additional validation considerations for certain AI-based systems.
I found this paper interesting because it exemplifies the developing awareness within the pharmacovigilance community of the need to discuss and document how ML models should be validated before being put into routine use. Importantly, the authors also emphasise the importance of aligning with regulators about the validation of AI solutions.
One part of the paper I believe will be useful for many pharmacovigilance professionals is the classification of automated pharmacovigilance systems into three categories – rule-based static, AI-based static, and AI-based dynamic. Rule-based systems rely on a fixed set of human-engineered rules and algorithms to automate certain processes. Static AI-based systems apply a fixed or locked pre-trained model to the data, and while they may be retrained, this does not happen automatically. Dynamic AI-based systems, on the other hand, continually adapt themselves on the basis of the data they receive. Currently, almost all pharmacovigilance systems are static, and the paper notes that work is needed to create validation frameworks suitable for future dynamic systems. I believe that such systems are still far from being used in practice.
The authors then propose a way to extend existing validation frameworks for static systems. They give a great overview of important elements for a master strategy for validating AI-based systems. At the heart of that is developing an understanding of what a system has – and has not – been trained to do. They also recommend a set of “good machine learning practices”.
From my perspective, one of the most important messages to emerge from this paper is the need for pharmacovigilance professionals and technical experts to collaborate. This especially resonates with my experience here at UMC, where we do most of our project work in inter- disciplinary teams. It has certainly been my experience that the more our data scientists and pharmacovigilance experts combine, the greater and more exciting the outcomes.
Read more